
Nonlinear measurements might be expected to confound, but do they belong in a statistical model hierarchy?
– By Rick Danielson Jr –
A statistician and physicist were discussing an experiment over a pot of tea, when this hypothetical exchange occurred: “I know we are working in the right units, but do you think that some of our measurements are nonlinear?” Puzzled, the physicist took a sip before responding, “Are you saying that individual measurements could be nonlinear? Is that a thing?” The statistician also paused to take a sip, “Well, Kruskal (1988) wrote about Mahalanobis (1947) using rulers with nonlinear increments. They were worried about people making correlated errors, but I guess that doesn’t apply to our instruments.” “No,” the physicist agreed, “not if we’re talking about repeated errors,” and the statistician added, “We do check our instruments.” Then the two finished each other’s thoughts again, “But if we were completely familiar with this experiment…” “…we wouldn’t be doing it!”
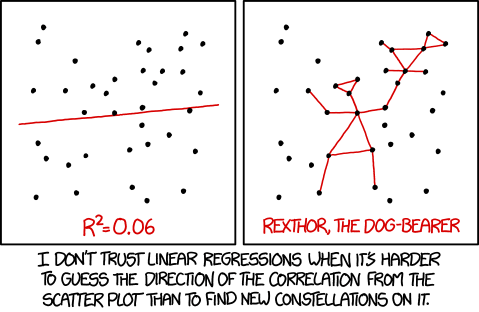
One purpose of this hypothetical exchange is to acknowledge that nonlinear measurements are unfamiliar. However, this exchange also illustrates a kind of model hierarchy. First, there is confirmation that a new idea exists (“is that a thing?”), then a decision about whether the idea is relevant (“No.”), and finally, an allowance of the unknown. In a sense, measures and models of the experiment are provided, respectively, by the statistician and physicist in different parts of the exchange. Together, they infer what actions to take if any. Perhaps the only notable thing is that this happens in just a few words.
Our other purpose is to question whether an admittedly ill-defined notion of nonlinear measurement might fit within a statistical model hierarchy. In addressing a wide audience, Salsburg (2017) emphasizes that the central tenant of statistical inquiry is that measurements = truth + error. In other words, measurements are linearly related to truth. Does this imply that our ruler has linear increments, or that we are using the most appropriate unit already? Instead, measurements that are taken to be equal to linear + nonlinear + unassociated, would seem to confound the central tenant, at least if truth = linear and error = unassociated. This is because the nonlinear part fits in neither category. While that might sound critical, what about our hypothetical exchange, which results in a conditional agreement to modify the experiment, but no action based on what is unknown or unfamiliar?
Perhaps we need to consider the central tenant as part of a hierarchy of models of varying complexity and familiarity. Such an idea is not exactly novel in biology and geophysics. Our hypothetical exchange can be offered as another accessible analogy. In other words, the decision to act is based the central tenant (a truth + error model), but the conversation certainly doesn’t end there. And nor should it end here. For instance, Mahalanobis (1947) predicts that as measurements become more precise, systematic bias should be easier to detect. It would be interesting to see if this prediction has held over the years since it was made, with ongoing advances in metrology, climate measurements, and their units.
Mahalanobis, P. C., 1947: Summary of a lecture on the combination of data from tests conducted at different laboratories (reported by J. Tucker Jr.). Amer. Soc. Test. Materials Bulletin, 144, 64–66.
This article was co-published with COSN and the SSC Liaison newsletter
Rick Danielson is currently a research scientist at the Bedford Institute of Oceanography, where he explores different measures of the marine and freshwater interface (mainly using Radarsat and WRFHydro). He was fairly consistently challenged by measurement modeling during four years at the Nansen Center in Bergen (Norway), two years each as a visiting scientist at the National Hurricane Center in Miami, and as a physical scientist at ECCC in Dorval and Halifax. Formative years were spent as a postdoc at Dalhousie University (with some enjoyable times in the local CMOS Centre) and as a grad student at McGill.