
Bias Correcting Surface Snow Water Equivalent Estimates using Machine Learning
-By Fraser King-
During Canada’s cold winters, snowpacks that aren’t consistently plowed or hovelled slowly grow in size and density. From a water-balance perspective, these snowpacks act as ephemeral water towers, waiting for spring temperatures to eventually warm them enough to melt en masse. This snowmelt-derived water is a critical contributor to local water budgets as it refills aquifers, and feeds nearby rivers and lakes. However, rapid snowmelt periods can quickly saturate the soil, leading to surface runoff and flooding. Snowmelt-derived flooding has become increasingly problematic across much of Canada in recent decades as global temperatures continue to rise, leading to millions of dollars in damage to local communities, and disruptions to regional ecosystem development and sustainability.
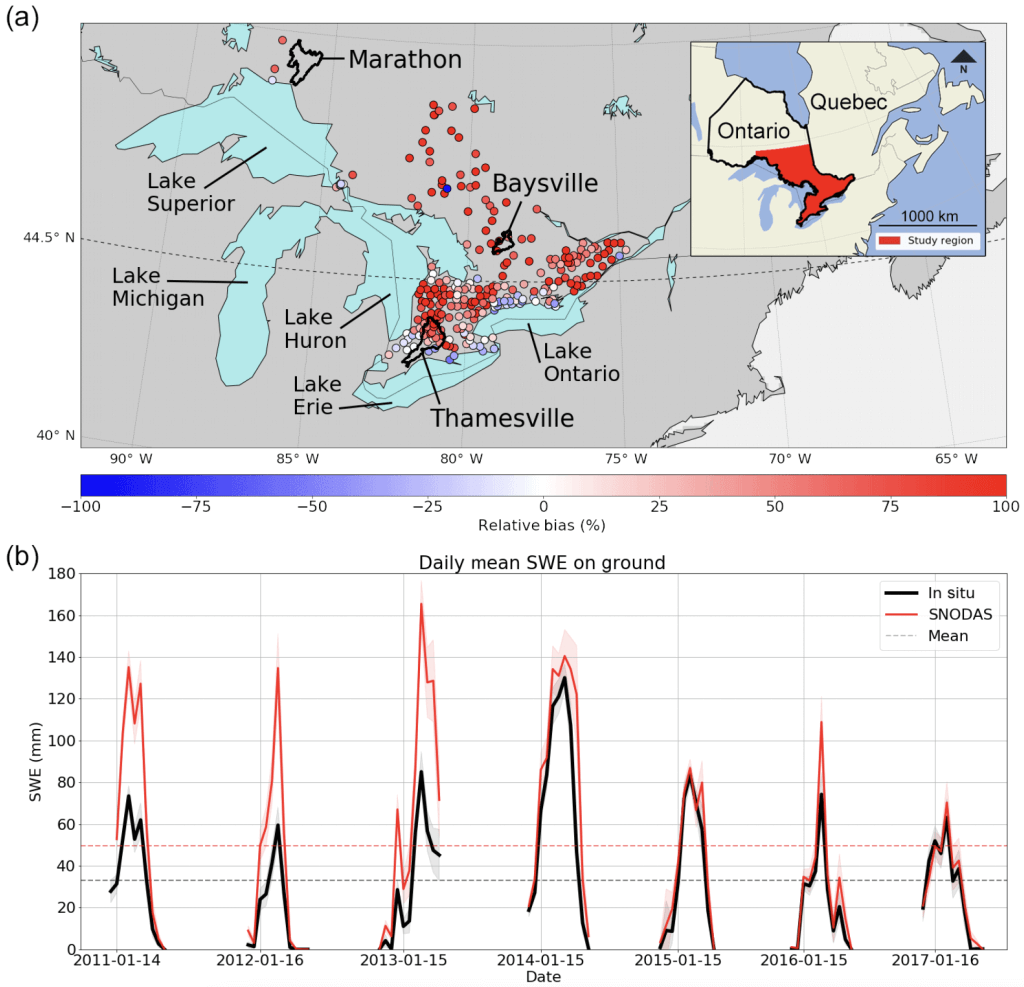
The ability to accurately quantify the amount of water stored in snow on the ground is therefore an important component in flood forecasting, allowing local governments to better prepare for, and mitigate, damages caused by future rapid snowmelt events. As discussed in another recent CMOS bulletin from Ross D. Brown, the number of snow-observing sites across Canada has dropped by over 50% since 1995, leaving large unobserved gaps across much of the country. Climate models and reanalysis products are powerful tools which can be used to fill these spatiotemporal gaps in observations, however no model is without bias, error and uncertainty, which limits our estimates of the true water content in a given snowpack.
In a paper submitted to Hydrology and Earth System Science in 2020, we address some of the aforementioned concerns surrounding model error by bias correcting snow water equivalent (SWE) estimates from the SNOw Data Assimilation System (SNODAS) gridded SWE product. SNODAS is a daily, 1 km modelling and data assimilation dataset produced by the National Oceanic and Atmospheric Administration (NOAA) National Weather Service’s Operational Hydrologic Remote Sensing Center. While this product was primarily developed for use across the continental United States, the northern portion of SNODAS overlaps with southern Ontario. Through a combination of the many sources of observations assimilated by SNODAS, and its complex, physically-based internal model, SNODAS produces some of the highest quality estimates of surface SWE across the region.
However, when compared with independent in situ measurements recorded by the Climate Research Division of Environment and Climate Change Canada (ECCC), SNODAS displays clear spatiotemporal biases in its SWE estimates across much of southern Ontario (Figure 1). Temporally, SNODAS exhibits a strong positive bias pre-2014 (a period which marks a distinct change in the known assimilated datasets), along with strong positive spatial biases as we move further inland, away from the US border.
To address these biases, we explored a suite of increasingly sophisticated statistical bias-correction methods, culminating in the application of a nonlinear machine learning (ML) technique which displayed the best overall skill. Instead of jumping directly into ML, we followed an “Occam’s razor” methodological approach by starting with simple, well validated and interpretable methods of bias correction like mean bias subtraction (MBS) and linear regression to develop a performance baseline. The idea being that if a simple method does nearly as well as a more sophisticated ML-based method, we should use the simpler, more explainable technique.
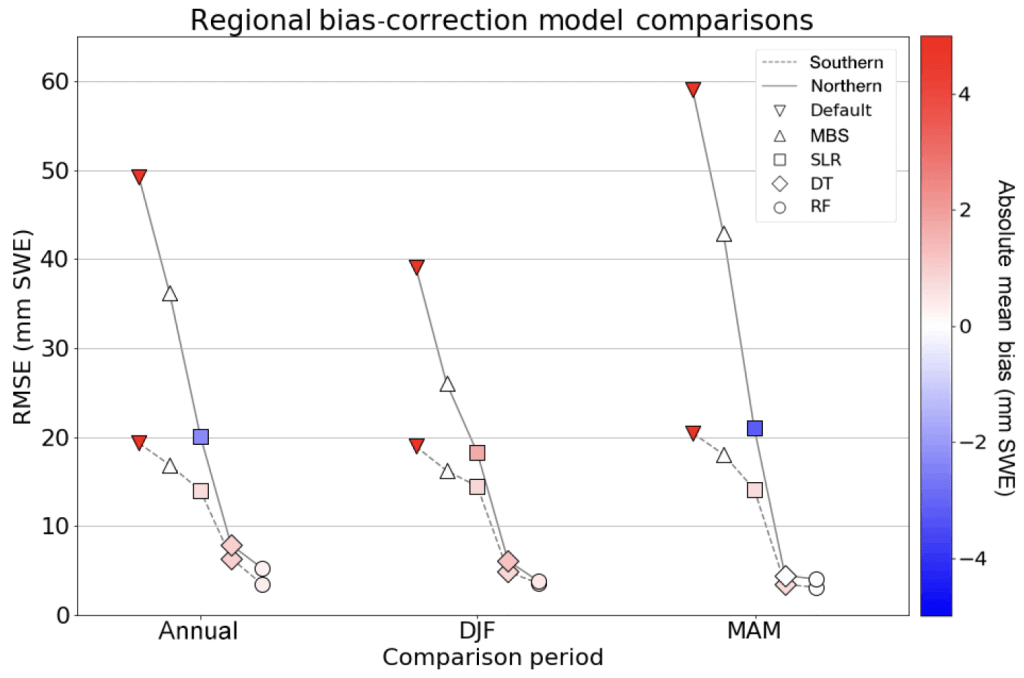
We evaluated four different models including the aforementioned MBS, a simple linear regression (SLR) model, decision tree (DT), and finally a random forest (RF). Each of these models were fit using the same training datasets over three periods
- December, January, February (DJF)
- March, April, May (MAM)
- DJF MAM (i.e. annual)
across two spatial domains (northern vs. southern Ontario). Each model was fit using a set of climate predictor variables (SNODAS SWE on ground, precipitation biases, surface temperature, elevation, year, and day of year) to model ECCC SWE on ground at 391 sites.
Our results indicated that the RF continually demonstrated the lowest overall RMSE and absolute mean bias over each period and across all regions (Figure 2). The overly simplistic MBS did an excellent job at removing the mean bias (by construction), however this was accomplished by overcorrecting the bias in some regions and under correcting it in others (resulting in the high RMSE for this method in Figure 2). The SLR fared better with a slightly lower overall RMSE, however these linear methods were unable to fully account for the nonlinear spatiotemporal bias from Figure 1. The best performing methods were the ML-based DT and RF, with the RF demonstrating improved performance annually (improved robustness).
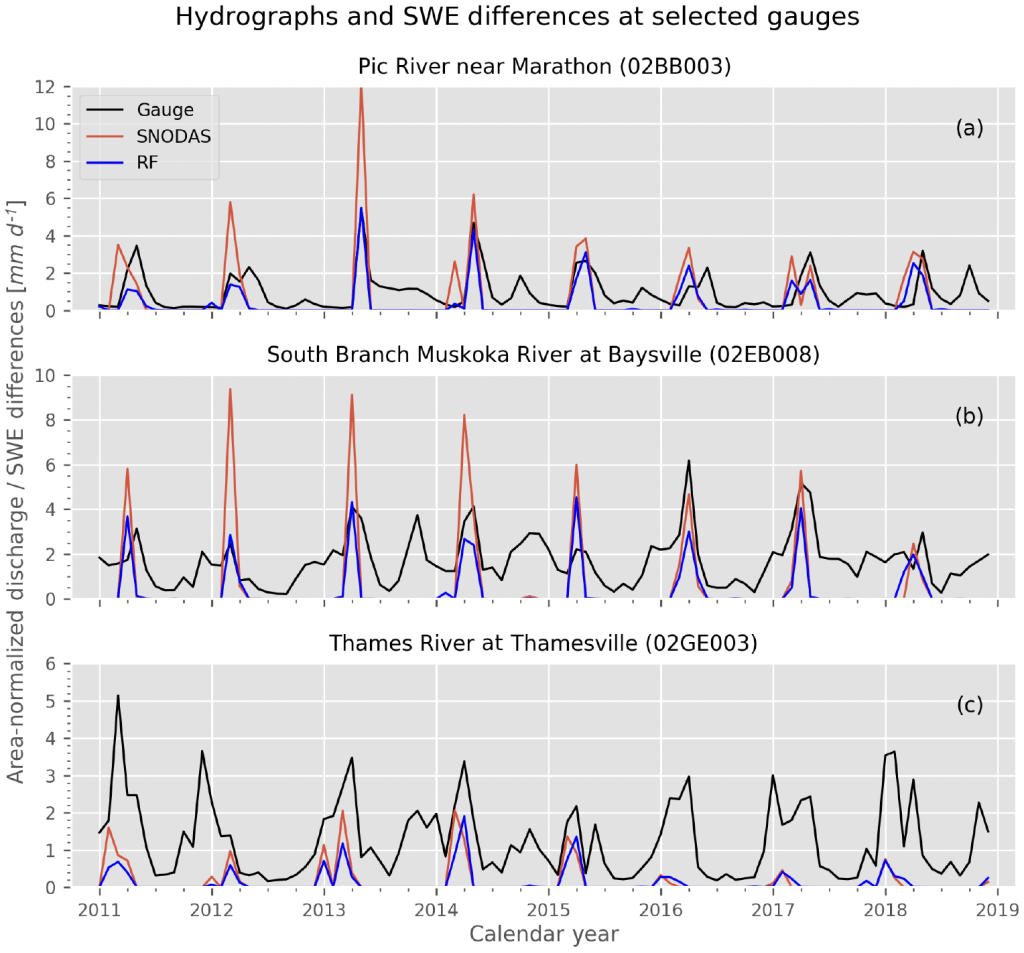
To further quantify the differences between bias-corrected and uncorrected SWE estimates, we also performed a simple water balance analysis across three watersheds in southern Ontario, anticipating that reductions in mean SWE would produce a more physically consistent fit with in situ melt measurements. Comparing monthly snow melt estimates (i.e. the negative SWE differences between consecutive monthly means from bias corrected and uncorrected SWE datasets) with area-normalized discharge across each basin (Figure 3), we found that the bias corrected RF-derived melt estimates were much closer in magnitude to in situ, and did not display the unphysical overestimation which was typical of SNODAS. These types of follow-up comparisons are incredibly useful methods for further validating the robustness of bias correction models like those explored in this work, and can be used to identify deficiencies which may be hidden upon first glance (e.g. preserving physical laws which are unknown to the ML model).
While the ML-based bias correction techniques applied here demonstrate good skill and a general robustness throughout the region, there are other options to choose from in the ML toolbox. In an upcoming study, we plan on evaluating some of these tools through a daily, ten-year Canada-wide bias correction of temperature, precipitation and radiation fields from the fifth-generation Canadian Regional Climate Model (CRCM5) (biases shown in Figure 4). With a much larger available sample in this follow-up project, we are able to experiment with more sophisticated neural network (NN) approaches for spatiotemporally bias correcting each climate variable. These bias corrected fields can then be used to drive land surface models and, in turn, bias correct surface snow estimates via a proxy correction of associated climate variables. When trained on the billions of available data points, early results suggest that NN approaches strongly outperform linear methods, and even beat out RF techniques for nonlinear biases like those present in surface temperature.
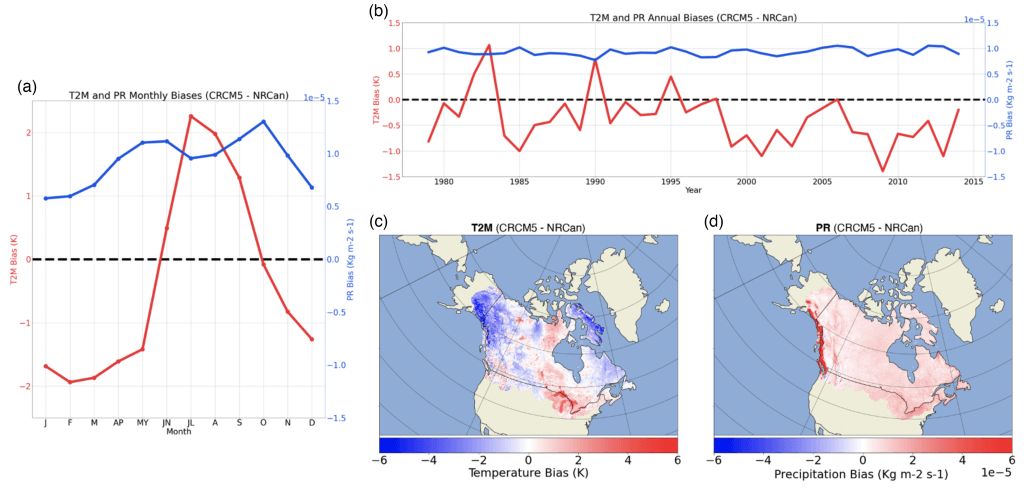
ML has been used across the Geosciences for decades, however, rapid advancements in computing resources, combined with petabytes of now easily accessible observational data, has allowed this field of research to flourish in recent years. While it can be appealing to immediately jump to ML for problems like downscaling or bias correction, we argue that this mindset may be problematic. The Occam’s razor approach to problems such as these provide researchers with additional opportunities to save on computational costs (i.e. avoid expensive model training/hyperparameterization phases), and to develop a more interpretable and explainable model. While we are incredibly optimistic about the future of ML (and especially deep learning) in the Geosciences, we also recommend that future researchers take care by starting with simple methods before digging into their respective machine learning toolboxes.
Fraser King completed his PhD in remote sensing and machine learning of precipitation at the University of Waterloo in December, 2022. He is now a post doctoral research fellow at the University of Michigan, developing machine learning-based snowfall retrieval algorithms and using surface and spaceborne radars to improve our understanding of hydrometeor particle microphysics.
fraser king, machine learning, snodas, snow water equivalent, snowpack